USING DYNAMIC BAYESIAN NETWORKS TO EVALUATE THE PHOTOVOLTAIC MODULES DEGRADATION PROCESS WITH ARTIFICIAL INTELLIGENCE
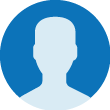
- Hybrid Renewable Energy Laboratory (HREL), University of Sciences, Techniques and Technologies of Bamako (Mali).
- Centre for Artificial Intelligence and Robotics of Mali (CIAR-Mali).
- Centre for Computer Science, Modeling and Simulation (CCMS), University of Sciences, Techniques and Technologies of Bamako (Mali).
- Modeling and Simulation Team (MGSI), Paris 8 University (France).
- Abstract
- Keywords
- Cite This Article as
- Corresponding Author
The performance of PV systems is highly dependent on climatic hazards (wind, dust, low sunshine, etc.). Some of these hazards can even accelerate its degradation process during its life cycle if nothing is done in terms of maintenance policy. This paper aims to model the degradation process of PV systems under environmental conditions. To do this, a system study is first performed to analyze the experimental data of the PV system in question according to the location of the site and simulated under the PVsyst software to extract the parameters of the study. In a second step, taking into account the Markovian approach, passing rules are established to design our dynamic Bayesian model. To this model, we have integrated a maintenance policy decision node and performance indicators in order to reproduce the degradation process in the real context and under stress. We have associated the decision node to enable AI integration through reinforcement learning on this node.The simulation results effectively reproduce the behavior of the PV system under environmental stress according to several scenarios (with or without IA). Furthermore, simulation allows us (a) to observe and validate the experimental values taken during the tests on the PV, (b) to see their availability increase with reinforcement learning compared to the case without learning. At the same time, we note that the increase in this availability leads to a relative decrease in income. The model allows to evaluate the performance of the system and propose the bestmaintenance policy configurations according to the input parameters (transition parameters, maintenance cost).
[Thierno Madani Sidibe, Fadaba Danioko, Mamadou Dansoko, Yacouba Issa Diakité, Sid-Ali Addouche and Abderrahman El Mhamedi (2025); USING DYNAMIC BAYESIAN NETWORKS TO EVALUATE THE PHOTOVOLTAIC MODULES DEGRADATION PROCESS WITH ARTIFICIAL INTELLIGENCE Int. J. of Adv. Res. (Jan). 872-884] (ISSN 2320-5407). www.journalijar.com
PhD student
Mali