CLASSIFICATION OF PULMONARY LESIONS USING GAN AND SEMI SUPERVISED GAN
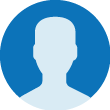
- Department of Electrical, Electronic and Systems Engineering, Faculty of Engineering & Built Environment, UniversitiKebangsaan Malaysia, Malaysia.
- Abstract
- Keywords
- Cite This Article as
- Corresponding Author
Lung cancer is a leading cause of cancer-related deaths, with early detection being important for effective treatment. This study investigates automated lung nodules classification using Generative Adversarial Networks (GANs) and semi-supervised GAN (SGAN), to address data scarcity issues in lung nodule classification. By generating synthetic lung nodule images, SGAN and conventional GAN models are evaluated on image quality, variation, and classification metrics, including accuracy, specificity, sensitivity, and test set loss. Although SGAN produces superior synthetic images, both models achieve comparable classification accuracy of 95%. GAN demonstrates slightly higher specificity and sensitivity. The results show that while SGAN generates enhanced synthetic images, the improvement does not result in better classification due to the grayscale nature of the images and effective feature extraction by the classifier.
[Amirul Haikal Abdullah, Siti Salasiah Mokri, Ahmad Abid Hakimi Md Salleh and QurratuAini Thaqifah Ithanin (2024); CLASSIFICATION OF PULMONARY LESIONS USING GAN AND SEMI SUPERVISED GAN Int. J. of Adv. Res. (Oct). 1582-1591] (ISSN 2320-5407). www.journalijar.com
Malaysia National University
Malaysia